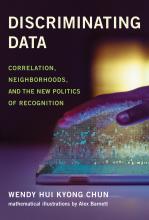
by Wendy Hui Kyong Chun
MIT PRESS, 2021, 344 PP.
HARDCOVER, $27.95
ISBN: 978-0-262-54852-6
In Discriminating Data, Wendy Hui Kyong Chun describes both how data are used to discriminate and how users must be discriminating in how they make use of data. Chun applies her cross-disciplinary academic background and identity as a first-generation Canadian immigrant to analyze the ideology of the Internet and surveillance culture. Chun draws on systems design engineering, media theory, and cultural theory to describe the past, present, and potential future created by digital technology, presenting the reader with a description of the biased roots and realities of a datafied world. Discriminating Data is split into four chapters: “Correlating Eugenics,” “Homophily, or the Swarming of the Segregated Neighborhood,” “Algorithmic Authenticity,” and “Recognizing Recognition.”
Analyzing examples that range from The Matrix to the math behind machine learning, Chun outlines the sinister origins of cyberspace, Internet culture, and artificial intelligence systems. Discriminating Data offers a philosophical perspective on critical theorists and technology writers, placing them within a constellation of other recent texts on the topic such as Weapons of Math Destruction, Race after Technology, and Data Feminism.1 Yet where other writers cite contemporary examples of the discriminatory nature of technology, Chun looks at foundational concepts to demonstrate that areas such as AI and machine learning have eugenicist roots and that network sciences are based on the same studies of homophily (attraction to the same) used to justify white flight and segregated housing in the 1950s.
While the book may seem at first to examine technology, it is in fact more a philosophical treatise on the theory of our current technological moment than on technology itself. Unlike other purely theoretical texts, however, Discriminating Data takes seriously the material reality of technology and the individuals who live mediated by it. The book’s power derives from the multidisciplinary themes and theories it braids together to describe the foundational ideology of the Internet and social networks as we know them today.
While deeply versed in several research areas, the book does not assume the same knowledge of its readers. With the aid of hand-drawn diagrams, the text does its best to reach an audience from a wide range of backgrounds such that the reader need not be an expert in all relevant areas. Such generality can become tedious at points to a reader already familiar with Chun’s core concepts, but it also ensures that the book paints a complete picture of its ideas. This is a difficult balance to maintain, and thus readers may find certain parts of the book too specific, while others seem too simplistic.
Based on studies ranging from Francis Galton’s criminal composites of 1878 to sociology of the 1950s, Chun outlines the academic research underpinning technologies we use today. She brings the reader into works that could be thought of as source code for today’s technosociety. Where research is limited, Chun turns to films such as The Matrix or the YouTube channel ContraPoints to explain how the initially liberal and liberatory ideals of Internet culture took a darker turn toward Donald Trump, conspiracy theories, and incel culture. In just one example of the long legacy that earlier discriminatory practices can have in the digital era, Chun highlights how the incel movement mirrors the racist pseudoscience of phrenology, with contemporary groups seeking to correlate physical characteristics such as skull shape with status.
By conducting a precise deconstruction of terms such as “authenticity,” Chun shows (in the mode of Jacques Derrida) their internal contradiction. Within the world of big data, social media, and the Internet, society risks entering a wonderland where authenticity is about style rather than content; love and hate are synonymous; and Fox News, the most watched television channel in the United States, complains about “mainstream media.” Chun cites how Donald Trump generated an artificial sense of authenticity, built through social media and reality TV, as evidence that social media encourages users to follow certain specific patterns to reproduce algorithmically determined authenticity. Algorithmic transparency ensures that predictable users can be targeted with advertising and recommendations to reproduce more and more of the same content. Donald Trump turned the 2016 US presidential election into an interactive reality TV program for the American public that flipped the script of his show, The Apprentice, by casting voters as judges with the power to “hire” or “fire” Trump himself. In this context, his “authenticity” became a product to buy and sell based on style rather than content.
While Discriminating Data describes the world as a dark place where outdated sciences continue to inform the present, the book is also hopeful, seeing the past as a warning rather than perdition. For example, we should sooner use AI and computer models to improve employee hiring practices today rather than to predict or create a set future. On climate change, Chun demonstrates that mathematical proxies, a source of much discrimination in online contexts, are neither innocent nor guilty but rather dangerous when used unquestioningly. With a detailed understanding of the ideological roots of machine learning and social networks, she creates a template for inquiry whenever users are promised simple answers. Ultimately, discrimination, polarization, and echo chambers are the goal, not an error. Chun asserts a narrative of liberation by pushing back against technological defaults and prescribed preferences, suggesting it is not by questioning or trying to fix technology but rather by understanding and resisting its biased founding ideologies that technology can enable a more liberatory politics instead of reproducing oppressions of the past. In her words, “To take on the twenty-first-century challenges of democracy, we need not a call to arms or a call for ‘new’ technologies—but a resolution to live freely, in difference” (254).
Discriminating Data is not a book I recommend as an introduction to the critical technology discourse. Its topics are wide-ranging, and it takes a detailed approach to explaining them. It is, however, an essential text for developing a theory of resistance to the oppressive tendencies of social networks, mass media, and surveillance AI across society.
—Bea Wohl, UNIVERSITY OF THE ARTS LONDON
1.Ruha Benjamin, “Race after Technology,” in Social Theory Re-wired: New Connections to Classical and Contemporary Perspectives, ed. Wesley Longhofer and Daniel Winchester (New York: Routledge, 2023), 405–15; Catherine D’Ignazio and Lauren F. Klein, Data Feminism (Cambridge, MA: MIT Press, 2023); Cathy O’Neil, Weapons of Math Destruction: How Big Data Increases Inequality and Threatens Democracy (New York: Penguin Random House, 2017).