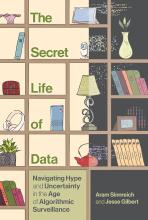
MIT PRESS, 2024, 312 PP.
HARDCOVER, $29.95
ISBN: 978-0-262-04881-1
In The Secret Life of Data, Aram Sinnreich and Jesse Gilbert set forth a difficult task for themselves. They aimed to write a book about algorithmic surveillance that carves a middle way between “sensationalistic or overtly partisan” work written for a general reader, on the one hand, and scholarship that “dives deeply into a specific aspect of the larger issue,” on the other (xiv). Readers of this journal will be no stranger to either type of book, but there are very few books that attempt to provide a ten-thousand-foot view while also offering some depth and theoretical sophistication. Sinnreich and Gilbert take on the task and succeed.
The Secret Life of Data begins from a relatively simple premise: “There is no limit to the amount and variety of data—and ultimately, knowledge—that may be produced from an object, event, or interaction, given enough time, distance, and computational power” (xii). The book piles up examples of how this premise plays out across a wide range of situations and technologies, and it does so by situating contemporary technologies in long historical trajectories, by interviewing twenty-nine different field experts, and by providing its own analyses of these technologies and their various cultural, ethical, and political implications. In addition, the book offers some key theoretical concepts that scholars across fields will find useful and that provide openings for future research.
One of those concepts is “algo-vision,” which the authors use to describe the internalization of the “obscure logic of the data systems that pervade our lives” (147). Algo-vision results in behavioral changes that serve the algorithm at the expense of nourishing user needs and desires. These shifts in behavior are harmful to individuals and groups, and they also make it much easier for the systems covered in this book to easily track people and activities—from machine learning and so-called generative artificial intelligence to the internet of things, predictive policing, the quantified self, and more. As we internalize these systems, we fill up databases that will eventually be put to various ends, some of which we can foresee and many of which we cannot. Opting out of algo-vision is essentially impossible at this point, but the authors also suggest that it might be put to interesting use as people figure out ways to resist and demystify systems. One example the authors offer is collaboration among Reddit users as they work to determine how and why YouTube’s content moderation systems are demonetizing and blocking certain channels (153).
The authors also theorize “triangulation,” a term they use to analyze systems that are multiperspectival and distributed. A key example is a smartphone app that helps support the Global Fireball Observatory (GFO) by encouraging users to point their cameras at the sky when they see a shooting star. In previous years, we might have lauded this as a “crowdsourced” effort, but that term has mostly been captured. Thus, “triangulation” (as well as another useful term the authors have developed, “crowdsourced stewardship”) offers a more complex and accurate account of what’s happening. The GFO application reimagines smartphones as something more than “selfie machines” and builds a map based on multiple perspectives, an effort that the authors contrast to something like Shot-Spotter, a predictive policing application that uses a distributed network of sensors to track the sounds of gunshots. If GFO is an effort at triangulation, the authors argue that ShotSpotter is essentially an example of Donna Haraway’s “God trick,” gathering data only from its own sensors and funneling that data to a centralized database that has “unilateral power to reshape data to fit a desired narrative or outcome” (169).1
To my mind, this idea of triangulation represents the most interesting aspect of the book. It is an example of how the authors argue for collective solutions to algorithmic surveillance. Such solutions are often extremely difficult to imagine from within a network of systems that encourage and thrive on individualized thinking. This more collective approach is the ethic underlying the authors’ research, and it is key to understanding how they stitch together far-ranging analyses of deepfakes, metadata, sensor networks, and unstructured data: “If we continue to relate to technology through the solipsistic lens of an individual user, rather than through the shared strength of our communities and cultures, we risk losing the fundamental autonomy and freedom of choice that are enshrined as human rights in modern democratic society” (159). As the authors suggest throughout the book, the choice is not between opting out or opting in but instead between building a just and equitable world based on solidarity and collectivity or buying the individualized snake oil sold by technology companies and their surrogates.
The authors present a mostly balanced view, but the book (correctly, in my view) focuses its attention on the dangers of surveillance technologies and how they threaten democracy, privacy, and liberty. It does present select positive examples of data-intensive systems achieving prosocial outcomes. For instance, the authors show how machine learning systems are being used to further historical research, including work that used DNA analysis to unequivocally prove that Thomas Jefferson fathered children with Sally Hemings. The authors also discuss examples of machine learning being used to reconstitute missing fragments of ancient sculptures. But these cases take a back seat to the many threats that algorithmic systems present. Further, the authors offer several ideas about how we might (again, collectively and not individually) address these threats by turning to existing research on data justice, data sovereignty, participatory design, algorithmic auditing, and the development of independent standards for algorithmic systems. Beyond these instrumental approaches to repair and design, the authors also gesture toward how we might reimagine possible futures with the help of “post-postapocalyptic fiction.” This subgenre can offer ways of imagining more just worlds by presenting us with “a glimpse into how people might begin to stitch it back together through forging bonds of trust and mutual aid when all of our social institutions have failed” (186).
One key audience for this book might be those outside the fields of information studies, media studies, or digital studies who are looking for an overview of the key questions regarding algorithmic surveillance. But for those within these fields, there is also useful material; in particular, the book provides theoretical concepts that researchers can take up, use, and extend. Sinnreich and Gilbert have written a nimble text that will be of use to a range of experts and nonexperts.
—James J. Brown, Jr., Rutgers University–Camden
1.Donna Haraway, “Situated Knowledges: The Science Question in Feminism and the Privilege of Partial Perspective,” Feminist Studies 14, no. 3 (1988): 575–99, https://doi.org/10.2307/3178066.